Tete Xiao
| Google Scholar | Github |
| Linkedin | CV | Twitter |
|
I co-founded Prompt AI and am currently serving as its CEO in the San Francisco Bay Area.
See more information from our press release.
I received a Ph.D. degree from UC Berkeley, advised by Prof. Trevor Darrell.
My research interests lie in the fields of computer vision, robotics and machine learning, with a focus on learning scalable representations via deep learning.
I was also affiliated with Facebook AI Research (FAIR),
where I was fortunate to work with Piotr Dollár and Ross Girshick.
Prior to UC Berkeley, I received a B.S. degree in Intelligence Science, summa cum laude, from Peking University (PKU) in 2019.
|
News
- [Oct. 2023] The press release about Prompt AI.
- [Oct. 2023] Prompt AI is mentioned in an article in WIRED Magazine.
- [Apr. 2023] Segment Anything (SAM) won the 2023 ICCV Best Paper Honorable Mention Award.
- [Apr. 2023] We released our latest project in vision: Segment Anything, one of very few computer vision systems that just works in the real world.
- [Mar. 2023] We released our latest project in robotics: Learning Humanoid Locomotion with Transformers, the first humanoid controlled by end-to-end neural networks.
- [Oct. 2022] We released the project: Real-World Robot Learning with Masked Visual Pre-training
- [Mar. 2022] We released the Masked Visual Pre-training for Motor Control (MVP)
---- show more ----
- [Oct. 2021] One paper accepted to NeurIPS21!
- [July 2021] Two papers accepted to ICCV21!
- [Jan. 2021] Two papers (including one selected for oral presentation) accepted to ICLR21!
- [Mar. 2020] Our work on compositional action recognition is accepted to CVPR20! Check out the project page with the new dataset "Something-else"!
- [July 2019] One paper on explainable human-object interaction is accepted to ICCV19!
- [May 2019] I will join the wonderful Berkeley Artificial Intelligence Research (BAIR) Lab as a Ph.D. student at the lovely UC Berkeley in August 2019. Go Bears!
- [Nov. 2018] ADE20K accepted to IJCV! We included a variety of interesting applications plus the study of synchronized batch norm for semantic segmentation. Check out the paper for more details!
- [July 2018] Two papers (including one oral) accepted to ECCV18! (Code is released!)
- [May 2018] Our work on contrastive samples in vision and language learning accepted to COLING18! (Paper and codes are released.)
- [Apr. 2018] A PyTorch implementation of scene parsing networks trained on ADE20K with SOTA performance is released in conjunction with MIT CSAIL. Check out our code, it's popular!
- [Feb. 2018] Two papers accepted to CVPR18!
- [Oct. 2017] As a team member of Megvii (Face++), we won the premier challenge of object detection - COCO and Places Challenges 2017: the 1st places of COCO Detection, COCO Keypoint and Places Instance Segmentation, as well as the 2nd place of COCO Instance Segmentation. I was invited to present at COCO & Places Joint Workshop at ICCV17 in Venice, Italy.
|
|
MegDet: A Large Mini-Batch Object Detector
Chao Peng*,
Tete Xiao*,
Zeming Li*,
Yuning Jiang,
Xiangyu Zhang,
Kai Jia,
Gang Yu,
Jian Sun
Conference on Computer Vision and Pattern Recognition (CVPR), 2018 (Spotlight)
*: equal contribution
| arXiv |
Scaling-up training of object detectors; winner of MSCOCO Challenge 2017.
|
Awards
- MSCOCO Challenge, 2017
- Snap Research Scholarship, 2019
- China National Scholarship, Peking Univsity
- Scholarship for the Outstanding Talented, Peking Univsity
- Schlumberger Scholarship, Peking Univsity
- Founder Group Scholarship, Peking Univsity
- Gold Medals, ACM International Collegiate Programming Contest (ACM-ICPC) Asia Regional, 2016 & 2017
- Bronze Medal, National Olympiad in Informatics (NOI), 2014
- Champion, Shandong Province Team Selection Contest for NOI, 2014
|
Service
|
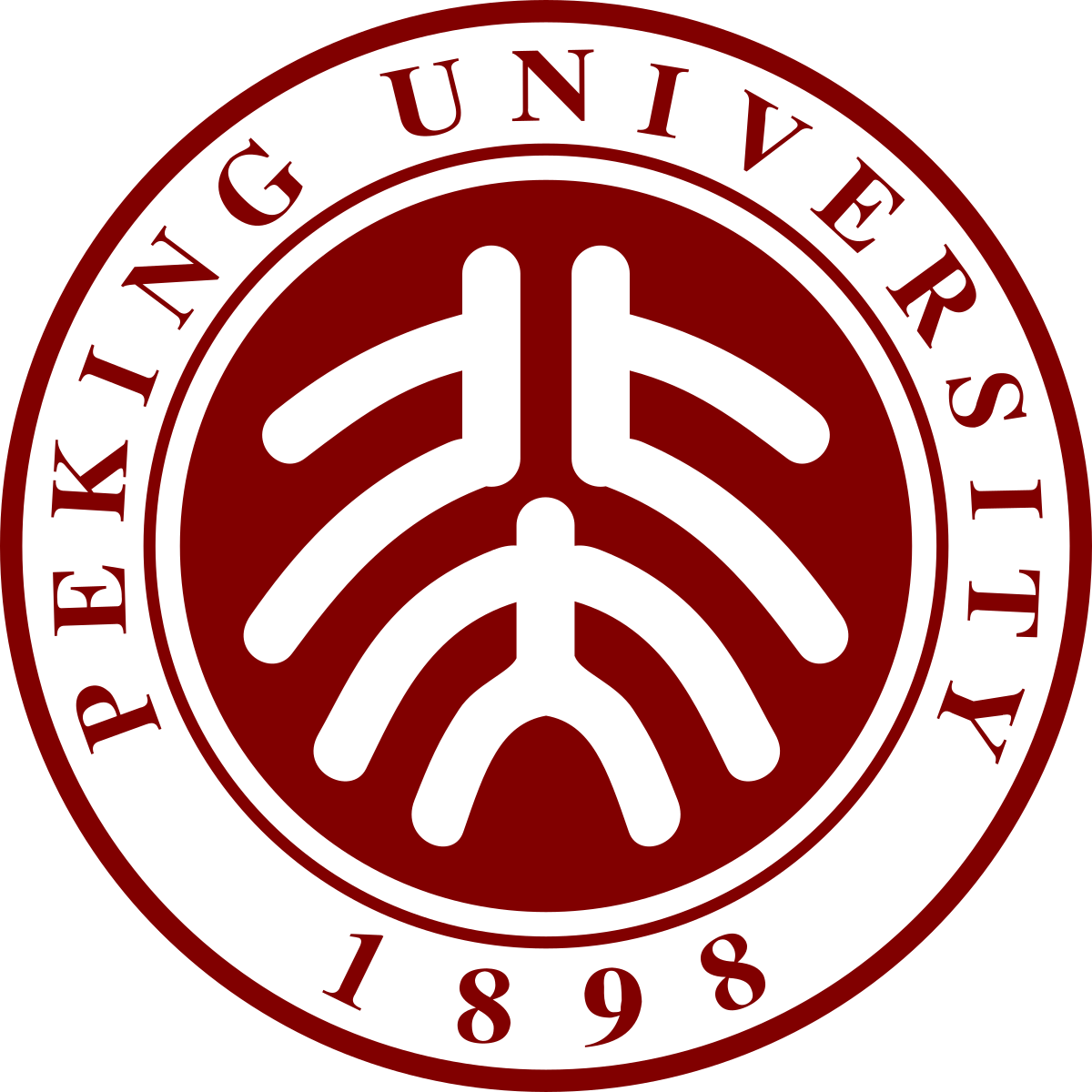 |
Teaching Faculty, Practice in Programming (17-18 spring)
Teaching Faculty, Artificial Intelligence and Computer Vision (18-19 spring)
|
Contact
Berkeley Artificial Intelligence Research Lab
Berkeley Way West, 2121 Berkeley Way
Berkeley, CA 94704
|
Website design: ✩ ✩
Avatar photo: taken in Jerusalem in July 2019 by my good friend Yingcheng Liu.
|
|